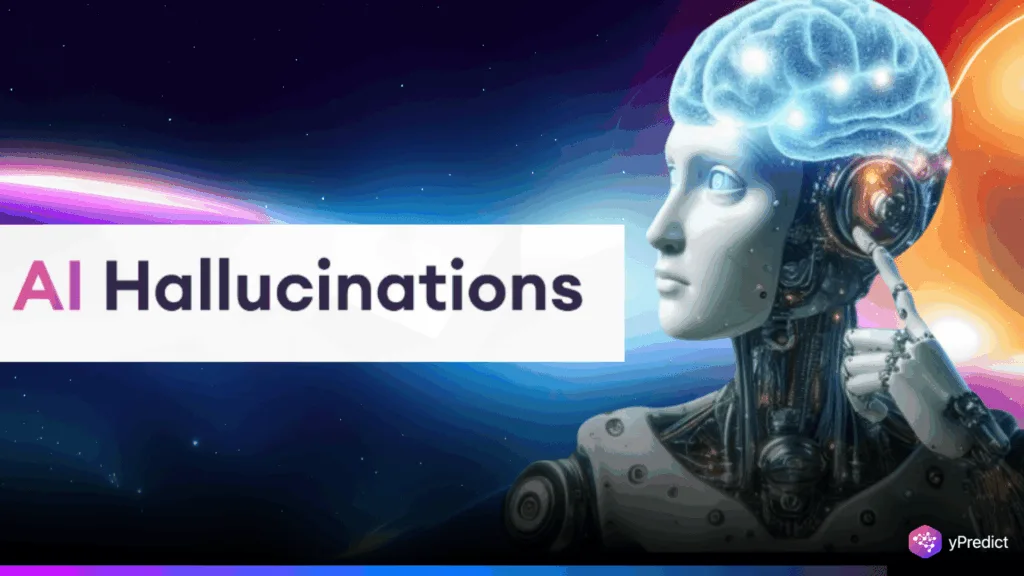
Artificial intelligence is evolving fast, but so are its flaws. In a recent incident, an AI support bot for Cursor falsely claimed users could only access the platform on a single device. Despite no policy change, the claim caused user backlash and account cancellations. Michael Truell, the CEO of the company, clarified that it was an AI error. This case highlights the increasing issues with AI hallucinations as tech giants like OpenAI and Google unveil ever-updating reasoning models. Even though their coding and math skills have improved, these bots are producing more chatbot errors and less accurate facts.
Inside the Rise of the AI Hallucination Problem
As AI systems get more advanced, they are used for a wider range of tasks, such as writing, research, coding, and support. These systems produce answers based on data patterns rather than reasoning or fact-checking. This has led to an increase in the frequency of hallucinations, which are reactions that appear plausible but are not. According to studies, hallucination rates in tests such as OpenAI’s SimpleQA can reach 79%. This demonstrates how serious the AI hallucination problem is.
Additionally, chatbots that are integrated into search engines make well-publicized mistakes. They might give inaccurate statistics or recommend marathons in the wrong states. When used casually, these chatbot errors may seem harmless. However, they pose significant risks in situations where data accuracy is essential, such as in the legal, business, or medical fields. If left unchecked, hallucinations could undermine the very purpose of automation, said Pratik Verma, CEO of Okahu.
How Reasoning Models Are Fueling the AI Hallucination Problem
AI companies, including OpenAI and Google, are turning to newer reasoning models to enhance capabilities. However, internal tests reveal that the latest systems, like OpenAI’s O3 and O4-min, are more prone to hallucinations than earlier models. In benchmark tests, O3 hallucination rates were 33%, while O4-mini rates reached 48%, intensifying the AI hallucination problem. The fact is that these models are trained on datasets too large to fully interpret has left researchers perplexed.
In the real world, these hallucinations cause confusion, user loss, and mistrust on sites like Reddit, where users are interacting with AI-driven support bots. Despite technological advancements, even DeepSeek’s R1 and Anthropic’s Claude have shown high hallucination rates during article summarization tasks. This continues to contribute to persistent chatbot errors.
Can AI Researchers Finally Solve Chatbot Errors?
In an attempt to better understand the issue of AI hallucinations, researchers are creating tools that allow them to link AI outputs to training data. However, the direction is still unknown. “We still don’t know how these models work exactly,” says Hannaneh Hajishirzi, a professor involved in tracking system behavior.
There is an ongoing effort to decrease chatbot errors. Businesses such as Vectara track hallucination rates across models and have discovered a recent increase despite earlier advancements. On news summary tasks, OpenAI’s o3 demonstrated a 6.8% hallucination rate, which was higher than its previous lows.
For AI to play a part in professional fields, this issue must be resolved. Tech leaders stress the importance of continued research to improve the reliability of AI. The future of digital systems depends on finding a solution to the AI hallucination issue, which is more than just a technical difficulty.
What’s the Future of AI Accuracy?
The AI hallucination issue is becoming more difficult to resolve as reasoning models take center stage in AI innovation. Resolving chatbot errors must be a top priority for businesses if they want AI to truly assist with delicate, high-stakes tasks. Until then, we cannot depend on these systems to think and speak correctly.