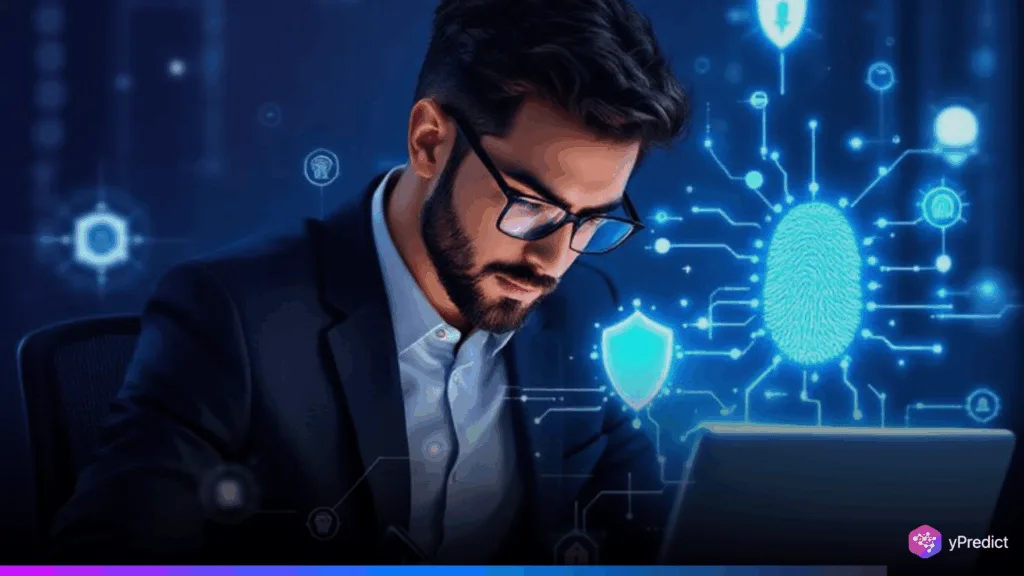
Despite promises of objectivity and fairness, a new academic study has found that many open-source AI hiring models exhibit strong gender bias, consistently favoring male candidates for higher-paying job roles. The research suggests that these tools, often relied upon to speed up resume screening in recruitment processes, may be perpetuating long-standing inequities rather than resolving them.
The study, conducted by Sugat Chaturvedi, assistant professor at Ahmedabad University, and Rochana Chaturvedi, a PhD candidate at the University of Illinois, evaluated how various large language models (LLMs) selected between equally qualified male and female candidates.
Using more than 300,000 English-language job listings from India’s National Career Services portal, the researchers prompted several LLMs to choose between anonymized resumes. The result: most models systematically preferred male applicants, particularly for higher-wage opportunities.
AI Models Reinforce Gender Stereotypes and Wage Disparities
The study highlights that AI systems not only replicate existing workplace bias but also recommend women for lower-paid roles. The researchers attribute this trend to deeply embedded gender patterns in training data and a reinforcement learning phase that favors so-called “agreeableness bias”, skewing the models to associate women with submissive or less assertive traits.
“This isn’t new with large language models,” said Melody Brue, VP and principal analyst at Moor Insights & Strategy. “Hiring bias has long existed, and since AI models are trained on massive datasets scraped from the web, they naturally absorb and reflect those same biases.”
The implications are stark. If employers continue to lean on these tools to streamline hiring, especially in tech, finance, and defense sectors, it may amplify existing gender inequities across industries.
Bias Varies Across AI Models
A recent study revealed striking differences in how various open-source AI models handle gender bias in hiring scenarios. Llama-3.1-8B-Instruct emerged as the most balanced, offering a 41% callback rate for female candidates and showing restraint by refusing to choose in nearly 6% of cases. In contrast, Gemma-2-9B-Instruct favored women more often (87.3% callback rate) but tended to recommend them for lower-paying roles, indicating a substantial wage penalty.
Gendered Job Patterns Persist and Reflect Broader Wage Gaps
The researchers further mapped the job ads to Standard Occupational Classification (SOC) categories. They found AI models more likely to recommend men for roles in male-dominated sectors (e.g., engineering, finance) and women for positions in female-dominated industries (e.g., education, HR).
Perhaps most troubling was the observed wage gap. Across nearly all models, women were directed toward lower-paying jobs, even when credentials matched male candidates. This finding underscores the need for greater transparency and auditing of AI-based hiring tools before widespread adoption.
Conclusion
The study reinforces growing concerns that AI tools unless carefully monitored and trained with balanced, bias-mitigated datasets, risk entrenching the very inequalities they are supposed to address. As companies increasingly depend on these systems to handle growing volumes of applicants, the call for ethical oversight, fairness testing, and inclusive design is more urgent than ever.