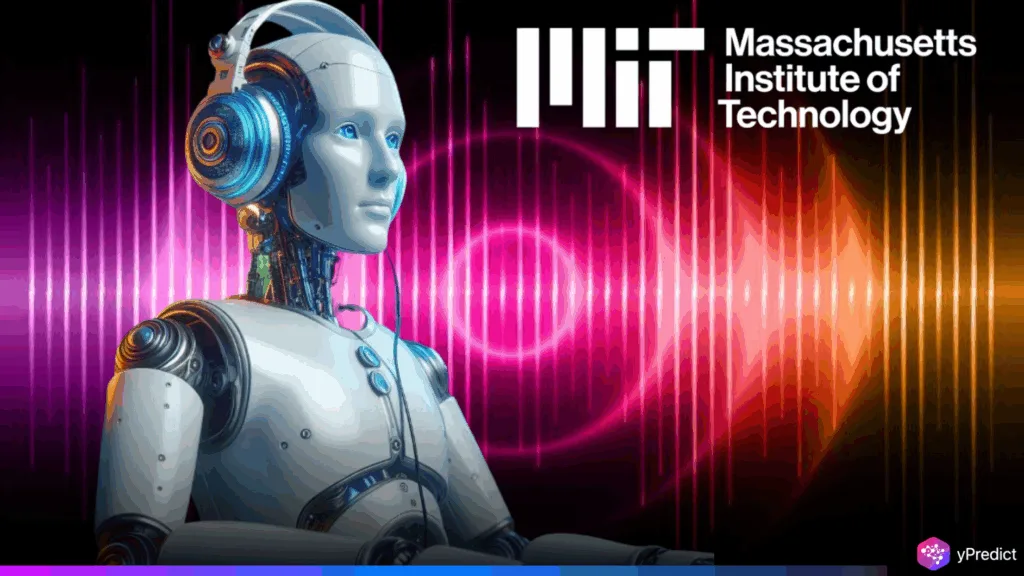
Researchers at MIT have created an innovative AI model that can automatically learn the connection between sound and visual input—without any labeled training data. This cutting-edge development has major implications for robotics, media, and audio-visual machine learning, bringing machines one step closer to perceiving the world like humans.
The AI system, CAV-MAE Sync, is an upgrade of MIT’s earlier model and uses self-supervised learning to associate what it hears with what it sees. The goal is to enable machines to detect sound-vision patterns independently, much like how humans naturally understand that a dog’s bark is linked to its image.
A Smarter Way to Sync Sight and Sound
Traditional AI systems often rely on large volumes of human-labeled data to connect audio and video. This method is slow, costly, and limited. CAV-MAE Sync takes a different path by learning from raw, unlabeled video clips.
The model improves over its predecessor by breaking audio into smaller time segments and matching each with corresponding video frames. This fine-grained approach makes it more accurate at identifying which sound goes with which part of a video—especially in cases where audio and visual cues are brief or scattered.
Dual-Token Architecture Powers Smarter AI Learning with Less Data
The team enhanced the AI model’s learning capabilities by implementing a dual-token structure made up of global and registered tokens. Global tokens are designed to compare and contrast video-audio pairs, enabling robust contrastive learning. On the other hand, register tokens assist the model in accurately reconstructing the original audio or video signals, promoting deep reconstructive learning. This innovative approach empowers the model to understand both relationships and fine-grained content with greater efficiency—eliminating the need for massive datasets or labor-intensive manual labeling.
Real-World Applications and What’s Next
The technology has real potential across industries. In robotics, such AI could help machines better understand their surroundings using sound and sight together. A service robot, for instance, could tell the difference between a door closing and a person speaking, improving its interaction with real-world environments.
In media and content management, it could allow users to search for video clips based on sound cues—making it easier to edit, tag, or retrieve scenes based on audio.
The researchers plan to extend the model’s abilities by integrating text processing, aiming for a unified system that can understand sight, sound, and language together. This could lay the foundation for multisensory AI assistants that respond more intelligently to human inputs.