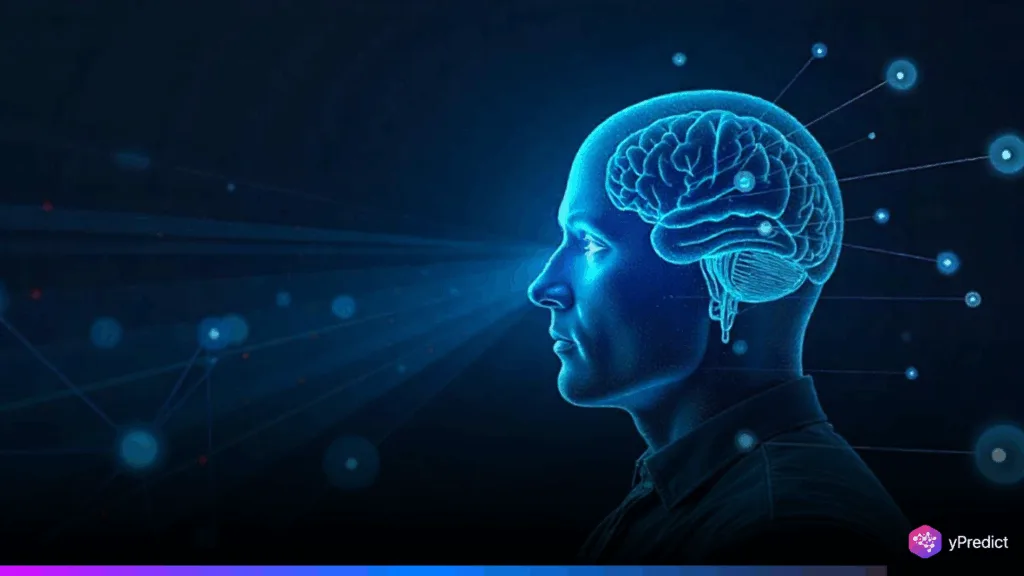
A report by Enkrypt AI has revealed alarming multimodal AI vulnerabilities. The report reveals how risky instructions hidden in images can bypass safety protocols in popular generative models. Under the direction of Sahil Agarwal, CEO of Enkrypt AI, the study describes how malicious actors might take advantage of these weaknesses.
The report draws attention to the possibility of producing risk outputs, such as chemical weapons instructions and child sexual abuse material (CSAM). Current AI security frameworks are seriously challenged by this discovery. Developers and companies utilizing these systems must give this their full attention right away.
Can Images Trigger Risks in AI Model Responses?
Enkrypt AI’s Multimodal Safety Report puts the spotlight on hidden risks within generative models that process both text and images. Using visual tricks like typography and steganography, the company showed through extensive testing that attackers could insert prompts into images. Despite being invisible to users, these prompts were able to get past filters in models like Mistral’s Pixtral-Large 25.02 and Pixtral-12 BB.
The research indicates that Pixtral models are 60 times more likely to produce text related to CSAM. They are also up to 40 times more likely to provide instructions about chemical, biological, radiological, and nuclear (CBRN) threats due to these multimodal AI vulnerabilities. In comparison, safer alternatives such as Anthropic’s Claude 3.7 Sonnet and OpenAI’s GPT-4o performed noticeably better in the same tests.
How Multimodal AI Vulnerabilities Are Exploited Today?
The research involved over 700 adversarial image-text pairs and followed a scientifically grounded method inspired by real-world abuse cases. The prompts evaluated how the models responded to various CSAM and CBRN scenarios. The researchers at Enkrypt AI discovered that even typographic text, words visible within images, was enough to bypass model filters. This finding raises serious concerns about AI security.
“Anyone with a basic image editor and internet access could perform the kinds of attacks we’ve demonstrated,” said Agarwal. Enkrypt emphasized that these issues are not theoretical. In addition to academic concerns, multimodal AI vulnerabilities pose real-world threats to public safety and business liability.
Models that are made publicly available are most vulnerable, particularly when they are implemented using tools like Mistral’s API or AWS Bedrock. AWS acknowledged safety as a “core principle,” but Mistral did not comment on the alarming results.
Fixing Multimodal AI Vulnerabilities with Real Safeguards
According to the report, developers should prioritize safety by implementing context-aware filters and putting in place real-time monitoring systems. Enkrypt advises implementing automated stress-testing procedures to stay up to date with changing threats. It also recommends “model risk cards” to openly communicate known vulnerabilities.
Agarwal underlined the necessity of context-aware filters that are able to understand operational and input context. These solutions are essential for the long-term development of generative models in public settings as well as for protecting against misuse.
In the future, Enkrypt AI intends to broaden testing to incorporate fresh and developing models. According to the organization, releasing these findings is essential to promoting responsible innovation and addressing security flaws. To protect against increasingly complex threats, proactive measures will be crucial as multimodal AI vulnerabilities develop.
What Will It Take to Secure AI?
The entire AI deployment ecosystem faces an immediate challenge as a result of the identification of these multimodal AI vulnerabilities. As the capabilities of generative technology increase, so does their vulnerability to abuse. If prompt action is not taken, the consequences may go well beyond technical problems. As a result, the industry needs to give these risks top priority.