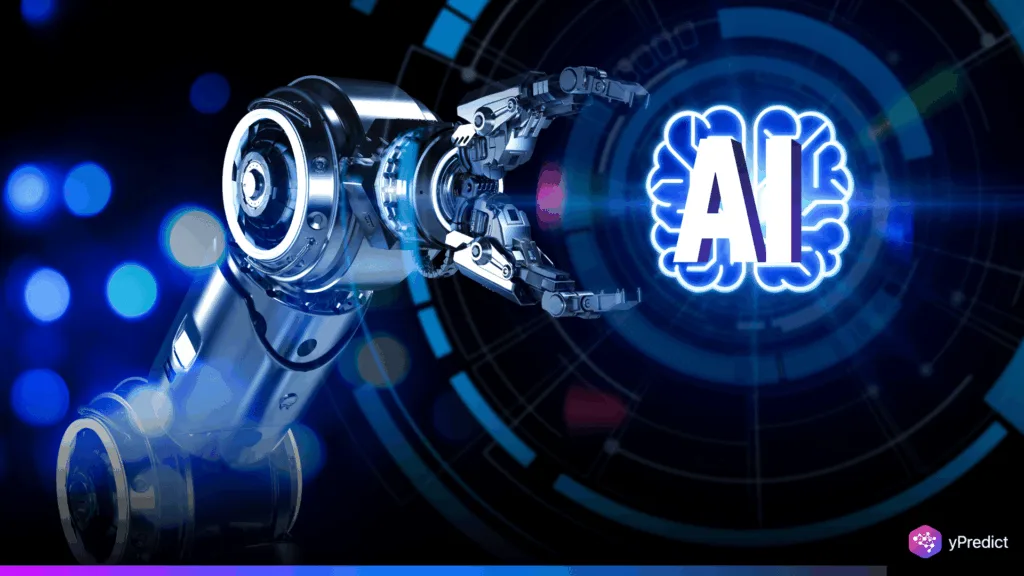
A recent analysis by the nonprofit AI research institute Epoch AI suggests that the era of rapid progress in reasoning AI models may be slowing. These models, such as OpenAI’s o3, have drawn notice recently for outperforming AI benchmarks.
However, the report cautions that high research costs and limits in reinforcement learning scalability pose challenges. Within a year, progress could slow down. Even with significant investments and improvements in AI performance, reasoning models may eventually plateau.
The Process Behind Smarter Reasoning AI Models
The ability of reasoning models to outperform traditional models in solving complicated problems makes them stand out. They achieve this by using more computing resources and advanced learning methods.
One notable difference is that reinforcement learning is a technique that enhances a model’s output by giving feedback on difficult problems. Although this method increases accuracy, it also makes model training more difficult and expensive.
For example, OpenAI’s o3 model significantly outperformed other models on recent AI benchmarks. It was trained with roughly ten times as much processing power as its predecessor, the O1.
The majority of this additional computing was probably used for reinforcement learning. However, as these systems get more powerful, they also get slower and cost more to operate. This makes it more difficult to continuously enhance AI performance without hitting limits.
Why Scaling AI Models Gets More Expensive
Epoch AI analyst Josh You notes that while more computing power is advantageous, this trend may not continue forever. According to his analysis, the advantages of reinforcement learning are increasing tenfold every few months. However, this rate of growth is not expected to continue.
Recently, OpenAI researcher Dan Roberts shared a post of his talk discussing reinforcement learning and more processing power. This is a reflection of the idea that future developments depend on models being improved through feedback. However, there is a limit to this tactic. The amount of computation that can be added before gains level out is limited.
Apart from computing limits, additional obstacles are emerging. There are significant overhead costs associated with researching and scaling reasoning AI models. These include increased energy demands, lengthy testing cycles, and specialized staffing. If such challenges continue, the pace of innovation in this field may fall short of industry expectations.
What’s Next for Reasoning AI Models Progress
By 2026, Epoch AI projects that advancements in reasoning AI models will probably follow more general AI trends. This suggests that the gap that currently exists between reasoning models and others may soon close. AI performance might not advance significantly year after year if the explosive growth of reinforcement learning slows.
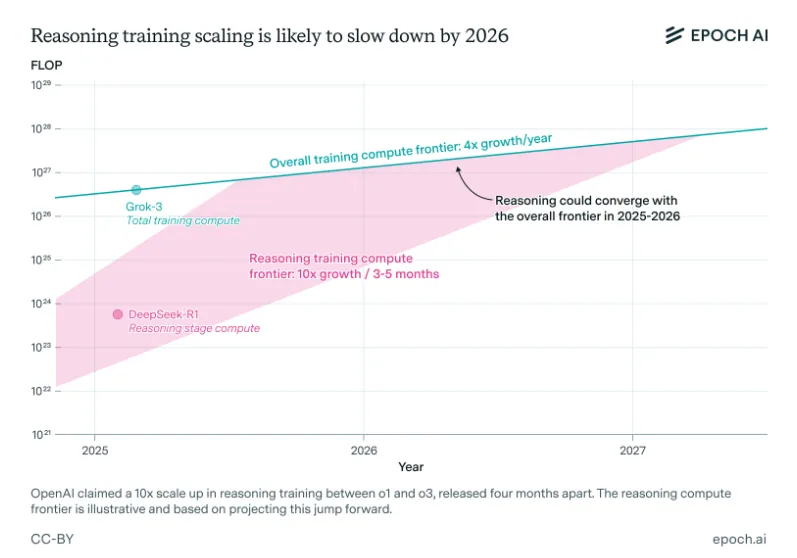
The report also notes that certain models of reasoning exhibit unanticipated flaws. Compared to some traditional models, they tend to “hallucinate” more. This problem raises additional doubts regarding the models’ dependability.
Organizations such as OpenAI are committed to investing more in training methods. However, it is evident that if computing and cost barriers are not addressed, breakthroughs may soon become more difficult to accomplish. It will be essential to monitor this development in order to comprehend the future of advanced AI systems.
Can AI Keep Evolving So Fast?
The AI sector has spent a lot of money developing reasoning AI models since they are the future of machine intelligence. However, if research overheads remain high and reinforcement learning reaches its limit, that future may not materialize as soon as expected. As 2026 approaches, the race to create smarter systems may enter a new phase where the focus is on more strategic innovation rather than rapid growth.