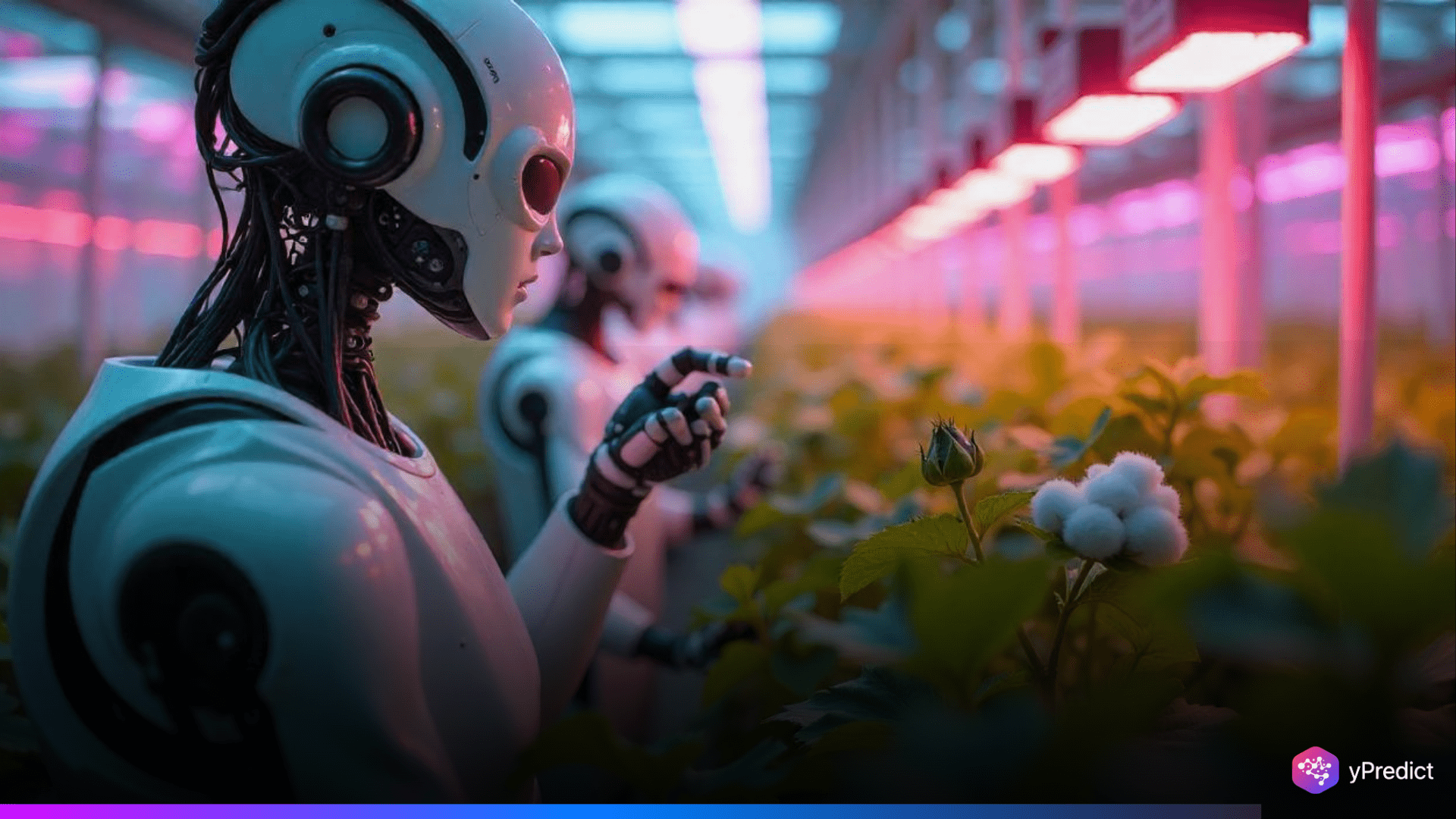
New AI model ESGAN speeds up crop breeding by reducing data needs 100x. Revolutionizing agriculture with self-learning computer vision tools.
A breakthrough in AI in agriculture could dramatically speed up crop research and breeding. Scientists at the University of Illinois Urbana-Champaign have developed a self-learning AI model that needs far less human supervision to analyze aerial crop images. Called the efficiently supervised generative and adversarial network (ESGAN), this system makes it much easier to track key plant traits like flowering time — a vital step for crop improvement and biofuel production.
ESGAN: A Smarter Way to Train AI for Farming
Traditionally, applying computer vision to farming required massive amounts of hand-labeled data. Researchers needed to manually tag thousands of images showing different crop features, making it slow and costly to build useful AI tools for agriculture.
However, ESGAN changes the game. It uses two AI models that “compete” with each other, learning to distinguish real crop images from fake ones generated by the system itself. Over time, the models get better without needing much human help. Tests showed that ESGAN can reduce the need for manually labeled images by 10 to 100 times compared to old methods.
How ESGAN Advances Crop Breeding Technology
The new approach was tested using Miscanthus grasses, a key crop for bioenergy. Flowering time is critical in breeding programs because it affects plant productivity and regional adaptability. Before ESGAN, tracking flowering time involved endless manual inspections of field trials.
Now, drones can capture images over large test fields, and ESGAN can automatically sort plants by their flowering status. This breakthrough makes crop breeding technology faster, cheaper, and more scalable. Instead of spending months labeling images, researchers can analyze massive datasets within weeks. The team, led by plant biologist Andrew Leakey and scientist Sebastian Varela, believes ESGAN will also work for other crops like maize, wheat, and rice, making it a game-changer for global agriculture.
Future of AI in Agriculture and Digital Farming
Looking ahead, the researchers plan to apply ESGAN to a multistate Miscanthus breeding project aiming to develop regionally adapted biofuel crops. They hope their tool will be widely adopted for other agricultural traits beyond flowering, boosting the overall efficiency of computer vision in farming.
The ESGAN model could also reduce costs in digital agriculture projects worldwide, helping farmers optimize crop yields, monitor soil health, and detect pests — all with minimal manual labor. As AI in agriculture continues to evolve, tools like ESGAN could make sustainable farming more achievable even on marginal lands.
Conclusion
The ESGAN approach marks a major leap forward in crop breeding technology. Slashing the need for human-labeled training data clears a path for broader, faster, and cheaper adoption of AI in farming. As researchers refine this tool across more crops and regions, AI in agriculture could soon become the norm — helping meet the world’s food, fuel, and environmental needs.