Microsoft revealed Phi-3 Mini recently, which is a spectacular language model with 3.8 billion parameters. Sparing its small size, it offers performance as comparable to models that are 10x larger, including but not limited to Mixtral 8x7B and GPT-3.5. Astoundingly, this was achieved through smart and efficient data-processing strategies with the model itself remaining unchanged.
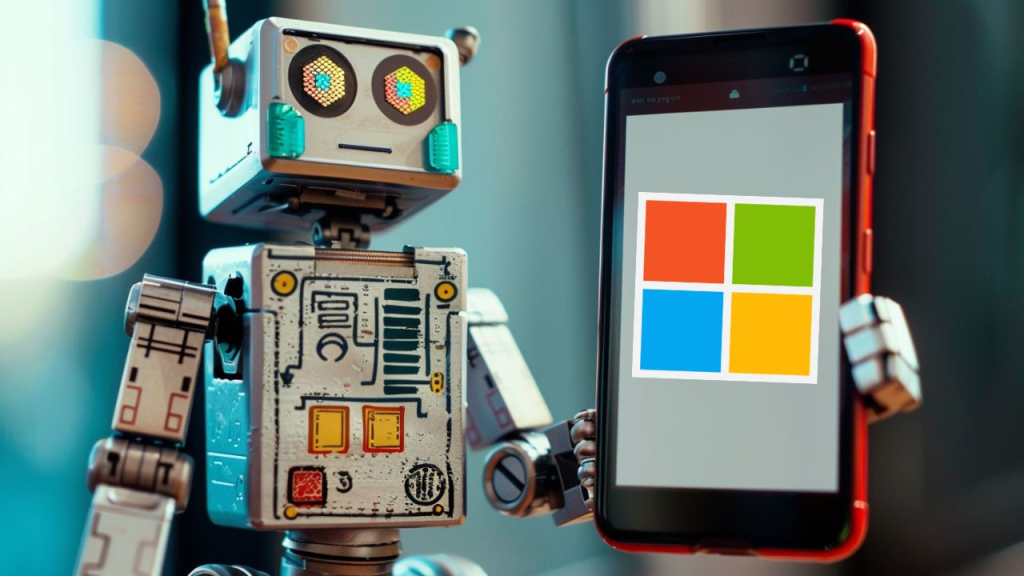
The main reason for Phi-3 Mini’s excellent performance is its training dataset strategy that has some improvements on the data foundation of its parent, Phi-2. The scientists accomplished this by capably filtering web data and integrating synthetic data crafted from larger language models. This new design led to the construction of a miniature model with an impressive expansion of features.
The PHI-3 Mini was also showcased as it excelled and inched on impressive scores of 69% on the MMLU benchmark and 8.38 on the MT-bench, which is worth noting almost of the same level of performance as models of much larger scale. Furthermore, alongside the progression stages of the training process, the model was tuned rigorously for both robustness, strong safety, and efficient management in replying to different dialogues.
One of the biggest advantages of the Phi-3 Mini is that is can perform directly on a smartphone. B6y way of quantization to 4-bit, the model’s memory space drastically reduced to as small as 1.8GB. This leap unlocked an iPhone 14 running on an A16 Bionic chip, enabling native on-board functionality and total offline operability. On the contrary, even with limited hardware capabilities at its disposal, Phi-3 Mini could still produce an output rate above 12 tokens per second.
Developers:✨Try @Microsoft's Phi-3 Mini with the 128K context window at https://t.co/1wkM1UY8BU ✨ https://t.co/W2lxJarKdh pic.twitter.com/1sm5HfrXOg
— NVIDIA AI Developer (@NVIDIAAIDev) April 23, 2024
Microsoft’s upcoming release roadmap includes two new additions to the Phi-3 series: Phi-3 “Small”, being 7 billion parameters or, Phi-3 “Medium”, being 14 billion parameters. Initial findings propose that these models are likely to become the new standards for smaller language models. Additionally, Phi-3 Medium achieved the acquisition of 78% on MMLU and 8.9 on MT-bench, where larger language models are superior.
Although Phi-3 Mini cannot own that much information in comparison to the larger models, its ability to make up for this gap by adding search functionality proves that it provides more flexibility to address this shortcoming. Consequently, the model’s capabilities were well-presented by integrating it with a search engine which enabled it to access necessary information in runtime.
While significant progress has been made, there are persistent challenges in tackling issues like factual inaccuracies, bias, and safety in language models. Nonetheless, through the implementation of meticulously curated training data, precise post-training adjustments, and insights gained from red-teaming, considerable strides have been taken to mitigate these concerns across various dimensions.